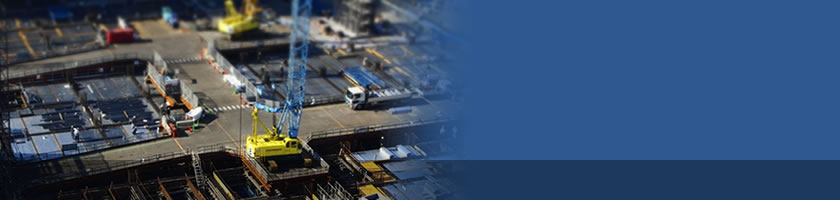







Vol.3 , No. 4, Publication Date: Sep. 3, 2018, Page: 87-95
[1] | Mohamed Nohair, Laboratory of Physical Chemistry & Bioorganic Chemistry, Energy, Interfacial Electrochemistry and Chemometrics, Faculty of Sciences and Technics, Mohammedia, Morocco. |
[2] | Mohssine El Marrakchi, Laboratory of Physical Chemistry & Bioorganic Chemistry, Energy, Interfacial Electrochemistry and Chemometrics, Faculty of Sciences and Technics, Mohammedia, Morocco. |
[3] | El Mati Khoumri, Laboratory of Physical Chemistry & Bioorganic Chemistry, Energy, Interfacial Electrochemistry and Chemometrics, Faculty of Sciences and Technics, Mohammedia, Morocco. |
[4] | Chaymae Jermouni, Laboratory of Physical Chemistry & Bioorganic Chemistry, Energy, Interfacial Electrochemistry and Chemometrics, Faculty of Sciences and Technics, Mohammedia, Morocco. |
[5] | Sara Azmi, Laboratory of Physical Chemistry & Bioorganic Chemistry, Energy, Interfacial Electrochemistry and Chemometrics, Faculty of Sciences and Technics, Mohammedia, Morocco. |
Physical processes influencing water temperature in a river are highly complex and uncertain, which makes it difficult to capture them in some form of deterministic model. Accurate forecasting of water temperature in a river is important, as it has implications on the quality of water and the lives that depend on it. Here we develop a model of forecasting which allows estimation and forecasting of water temperature at short and middle term, It intends to forecast the water temperature of days (t+i, i=1,2…), t is the current time. Due the strong dependence between water temperature at the current time and those for the past, the projected model builds easily itself, by investigating, for each stage of forecasting, the function relating input and output relationships. For this, a multi-step-ahead forecasting model based on the neural networks with the Bayesian regularization technique, is formulated for establishing linkages between water temperature and influencing variables. The results show that the elaborated model is robust and reliable and gives good results. It allows us to forecast the water temperature with high success. To test the ability of the model for the prediction, the observed data of the average daily water temperature during a period of five years (1998-2002) is considered for analysis. The first three years serve for the training and the remaining for the test. The model produced a standard coefficient R about 98, while the standard deviation s does not exceeds 0.6°C. We noticed there are a few cases presenting an error between 1 and 1.5°C (On average three cases for all steps of forecasting).
Keywords
Water Temperature, Small Stream, Forecasting, Neural Networks Analysis, Bayesian Regularisation
Reference
[01] | Coulibaly, P., Anctil, F. & Bobeé, B. (1999) Prévision hydrologique par réseaux de neurones artificiels: Etat de l’art. Can. J. Civ. Engrg., 26 (3), 293–304. |
[02] | Hipel, K. W. & McLeod, A. I. (1994) Time series modelling of water resources and environmental systems. Elsevier, Hollande. |
[03] | Thirumalaiah, K. & Deo, M. C. (2000) Hydrological forecasting using neural networks. J. Hydrologic Eng., 5 (2), 180-189 |
[04] | WMO, (1994) Guide to hydrological practices. WMO-No. 168, Genève, Suisse. |
[05] | Garner G, Hannah DM, Sadler JP & Orr HG (2014) River temperature regimes of England and Wales: spatial patterns, inter-annual variability and climatic sensitivity. Hydrological Processes 28 (22): 5583-5598 |
[06] | Cole JC, Maloney KO, Schmid M & McKenna JE (2014) Developing and testing temperature models for regulated systems: A case study on the Upper Delaware River. Journal of Hydrology 519: 588-598. |
[07] | Dreyfus, G., Martinez, J., Samuelides, M., Gordon, M., Badran, F., Thiria, S. & Hérault, L. (2002) Réseaux de neurones - Méthodologie et applications. Edition Eyrolles. |
[08] | Govindaraju, R. S. & Rao, A. R. (2000) Artificial Neural Networks in Hydrology. Springer; 1 edition. |
[09] | Liang, R. H. & Hsu, Y. Y. (1994) Scheduling of hydroelectric generations using artificial neural networks. IEEE Proceedings, Generation Transmission and Distribution, 141 (5), 452–458. |
[10] | Chang, F. J., Chiang, Y. M. & Chang, L. C. (2007) Multi-step-ahead neural networks for flood forecasting / Réseaux de neurones à échéances multiples pour la prévision de crue. Hydrol. Sci. J., 52, 114-130. |
[11] | Birikundavyi, S., Labib, R., Trung, H. T. & Rousselle, J. (2002) Performance of neural networks in daily streamflow forecasting. J. Hydrologic Eng., 7 (5), 392-398. |
[12] | Maier, H. R. & Dandy, G. C. (1996) The use of artificial neural networks for the prediction of water quality parameters. Water Resour. Res., 32 (4), 1013–1022. |
[13] | Zhang, Q. & Stanley, S. J. (1997) Forecasting raw-water quality parameters for the north saskatchewan river by neural network modelling. Water Resour. Res., 31 (9), 2340–2350. |
[14] | Zhang, S. P., Watanabe, H. & Yamada, R. (1994) Prediction of daily water demands by neural networks In: Stochastic and statistical methods in hydrology and environmental engineering. 3. (ed. by K. W. Hipel, McLeod, A. I. Panu, U.S. & Singh, V. P.) 217–227, Kluwer Academic Publishers, Dortrecht, Hollande. |
[15] | Sezin Tokar, A. & Momcilo, M. (2000) Precipitation-runoff modelling using artificial neural networks and conceptual models. J. Hydrologic Eng., 5 (2), 156-161. |
[16] | Coulibaly, P., Anctil, F. & Bobeé, B. (2001) Multivariate reservoir inflow forecasting using temporal neural networks. J. Hydrologic Eng., 6 (5), 367-376. |
[17] | Bélanger, M., El-Jabi, N., Caissie, D., Ashkar, F. et Ribi, J.-M. (2005) Estimation de la température de l’eau en rivière en utilisant les réseaux de neurones et la régression linéaire multiple. Revue des sciences de l’eau, 18 (3): 403-421. |
[18] | Menchero, A., Diez, R. M., Insua, D. R. & Müller, P. (2005) Bayesian analysis of nonlinear autoregression models based on neural networks. Neural Computation, 17, 453-485. |
[19] | Titterington, D. M. (2004) Bayesian methods for neural networks and related models. Statist. Sci., 19 (1), 128-139. |
[20] | MacKay, D. J. C. (1992) Bayesian interpolation. Neural Computation, 4, 415-447. |
[21] | Rudolph, M. (1997) On topology, size and generalization of non-linear feed-forward neural networks” Neurocomputing, 16, 1–22. |
[22] | Haykin, S. (1994) Neural networks: a comprehensive foundation, Mac-Millan, New York. |
[23] | Hassoum, M. H. (1995). Fundamentals of artificial neural networks. Eds., MIT Press, Cambridge. |
[24] | Hagan, M. T. & Menhaj, M. B. (1994) Training feedforward networks with the Marquardt algorithm. IEEE Trans. Neural Networks, 5 (6): 989-993. |
[25] | MATLAB 7.0, The MathWorks, Inc., Natick, MA. 2002. |
[26] | Cluis, D. A. (1972) Relationship between stream water temperature and ambient air temperature – A simple autoregressive model for mean daily stream water temperature fluctuations. Nordic Hydrology, 3 (2), 65-71. |
[27] | Caissie, D., El-Jabi, N. & Satish, M. G. (2001) Modelling of maximum daily water temperatures in small stream using air temperature. J. hydrology, 251, 14-28. |
[28] | Morrill, J. C., Bales, R. C. et Conklin, M. H. (2005). Estimating stream temperature from air temperature: Implications for future water quality. Journal of Environmental Engineering-Asce, 131 (1): 139-146. |
[29] | Mohseni, O. & Stefan, H. G. (1999) Stream temperature/air temperature relationship: a physical interpretation. J. Hydrology, 218, 128-141. |
[30] | Moatar, F., Bustillo, V., Ducharne, A., Thierry, D., Sauquet, E. et Vidal, J. F. (2010). ICC-HYDROQUAL– Impact du Changement Climatique sur l’hydrosystème Loire Hydrologie, Régime thermique, qualité des eaux. Plan Loire Grandeur Nature. |
[31] | Ducharne, A. (2008) Importance of stream temperature to climate change impact on water quality. Hydrology and Earth System Sciences, 12 (3): 797-810. |
[32] | Ficklin DL, Stewart IT & Maurer EP (2013) Effects of climate change on stream temperature, dissolved oxygen, and sediment concentration in the Sierra Nevada in California. Water Resources Research 49 (5): 2765-2782. |
[33] | Hopper, P. H. (2001) Applying the scientific method to small catchement studies: a review of the Panola Mountain experience. Hydrol. Processes, 15, 2019-2050. |
[34] | Burns, J. A. & Whitesides, G. M. (1993) Feed-Forwards Neural Networks in chemistry: Mathematical Systems For classification and Pattern Recognition. Chem. Rev., 93, 8, 2591-2601. |
[35] | Bishop, C. M. (1995) Neural Networks for Pattern Recognition; Oxford, University Press. |